
With low growth and high certainties, manufacturers are often forced to squeeze every asset for maximum value. Most manufacturers have already made the most obvious changes to streamline their operations, using traditional methods to eke as much productivity out of their supply chains and plants as possible. However, most manufacturers have not optimised their own data to obtain insights.
Process industries generate enormous volumes of data, but many have failed to make use of this mountain of potential intelligence. Analytics generates value only when it changes business decisions or actions for manufacturers. This means how data is consumed rather than how it is produced.
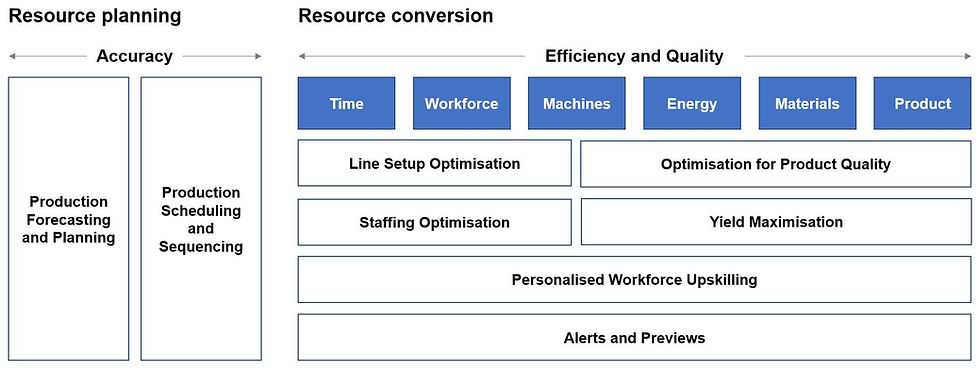
To do manufacturing analytics well, resource planning must be as accurate as possible (see figure above), then the resource conversion can be planned. It is about configuring the manufacturing process for optimal efficiency, throughput and quality.
Using data from sensors to define optimal point for production and cost
With predictive analytics, sensors are embedded in machines and advanced algorithms comb through data sets to uncover trends and issues faster. There can be advanced modelling to define an optimal setup point (e.g. sales mix, value allocation, procurement mix) to maximise profit per hour generation.
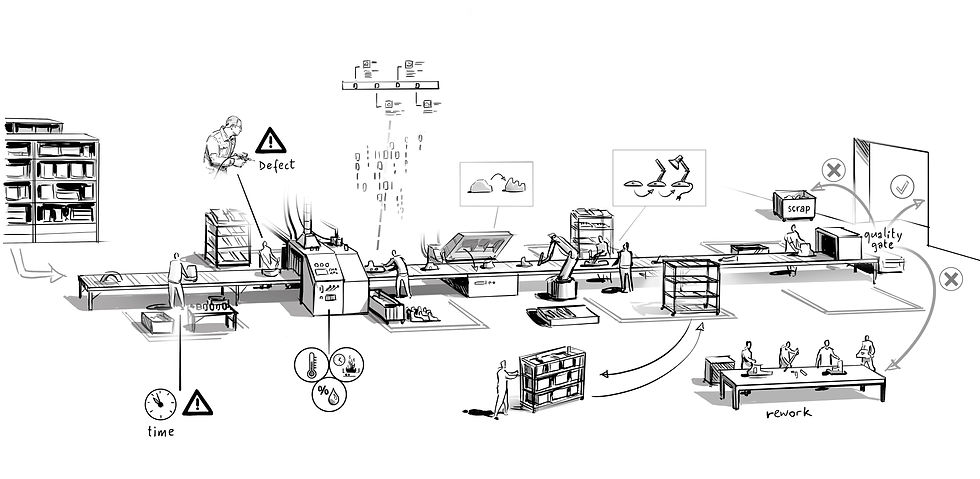
Using data captured from sensors, along with advanced-analytics tools, industrial companies can deploy self-learning models that simulate the expected value and cost of individual processes and even entire factories on a continuous basis.
There can be minimal switching production from one product to another, known in the industry as making a “changeover”, as this can involve the time- and resource-consuming exchange or re-configuration of machines, tools and production lines. This unproductive time can account for several percentage points of reduced machine utilization and line downtime.
Additionally, in some factories, the rate of defective parts is so high — as much as 30 percent — that the factory spent almost as much money making defective products as it did making quality products. Clearly, understanding and correcting the causes behind defective parts can provide significant cost savings.
Analytics helps push manufacturing productivity to the max. It determine how much of and when each resource will be needed to make a certain quantity of product. For example, it can be used to maximise machine utilisation and save energy consumption.
Maintenance can be performed more effectively
Predictive maintenance is a step forward from preventive maintenance. It aims to improve production efficiency and machinery efficiency. It identifies patterns that lead to a failure, and integrate them as new rules into the proactive workflows.
Predictive maintenance systems can then gather historical data (structured and unstructured, machine- and non-machine-based) to generate insights that can’t be observed with conventional techniques.
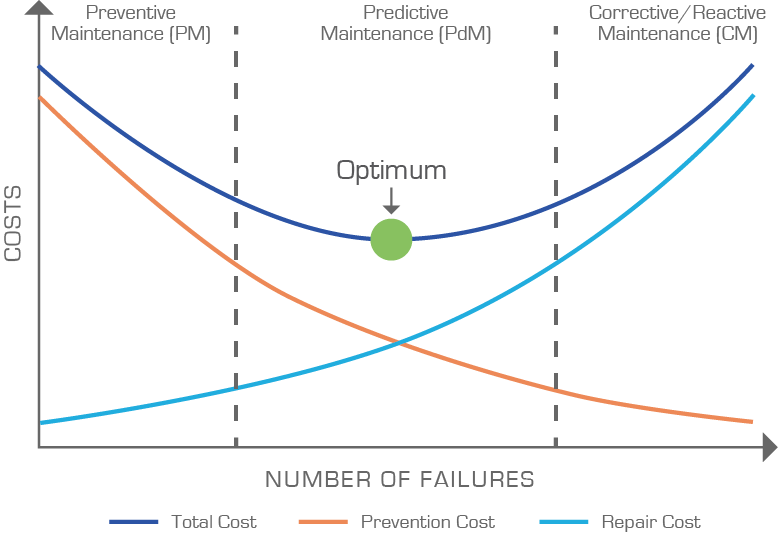
Imagine you are able to predict or identify problematic hardware before anything even goes wrong. You could effectively take action to repair, service or replace the equipment, eliminating the need for downtime entirely. Downtime is a major factor that hinders the attainment of manufacturing efficiency.
McKinsey found that predictive maintenance typically reduces machine downtime by 30 to 50 percent and increases machine life by 20 to 40 percent. This significantly increases profit margins for companies, where the ill effects of downtime and the related costs can be eliminated.
Getting from here to there with change management
Change management is important. When implementing analytics, manufacturers must consider the human aspect to have employees buy-in to the tool. If there is no buy-in, they will reject the tools as a threat rather than an opportunity, and manufacturers will be unable to advance from data to insight to actual business benefit.
Both formal and informal effort is required to achieve buy-in.

Process design and training is also important to instill new practical approaches and give people the knowledge and cultural support they need for the change. Many believe that the process will be more efficient if fewer people are involved in planning. But that is not true. Although it may take longer in the beginning, ensuring broad involvement saves untold headaches later on.
You must also be aware of change fatigue where people feel pressured to make too many changes/ transitions at once. Change must be ensured to be able to be sustained over time where targets are realistic.
By prioritizing high-value use cases, the advanced analytics program can often be at least partly self-funding. Deploying analytics is not an one-off exercise, these analytics will need to be repeatedly deployed to achieve the desired outcomes.
With the introduction of analytics tools, companies must learn to actively manage the cultural and organizational changes that follow, which is critical to implementing analytics.
What do you think about using analytics in your manufacturing processes? Share with us by leaving us a comment. If you require more information or assistance on analytics, contact us. Subscribe to our newsletter for regular feeds.
Did you find this blog post helpful? Share the post! Have feedback or other ideas? We'd love to hear from you.
References
Cerasis, Predictive analytics in manufacturing, https://cerasis.com/predictive-analytics-in-manufacturing/
IVC Technologies, Reactive, Preventive and Predictive Maintenance, https://ivctechnologies.com/2017/08/29/reactive-preventive-predictive-maintenance/, published 29 August 2017
Strategy + Business, 10 Principles of Leading Change Management, https://www.strategy-business.com/article/00255?gko=6c601, published 6 June 2014
Comments